A new deep-learning AI algorithm, developed as part of a study by the University of Cambridge, can identify and classify ‘Hard to Decarbonise’ houses (energy-wasting homes) with 90% accuracy.
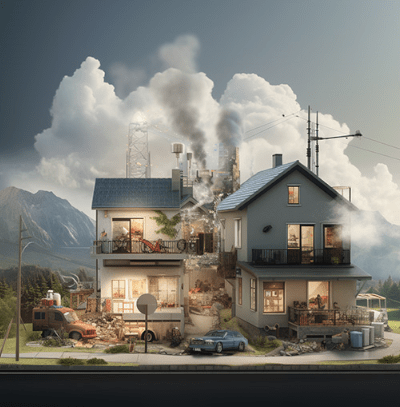
What Are ‘Hard-to-Decarbonise’ Houses?
Hard-to-Decarbonise (HtD) houses/buildings are really a subset of residential structures that present unique challenges in reducing carbon emissions due to their design, old age, construction, location, or the behaviours of occupants.
Why Are They Such A Problem?
These buildings are significant because they account for a sizable portion (roughly a quarter) of all homes and are responsible for over 25 per cent of direct residential sector emissions. As the urban population looks set to swell, ensuring these buildings’ sustainability has, therefore, become critical to meeting the global carbon reduction goals.
Why Hasn’t This Problem Been Tackled Effectively So Far?
The effort to decarbonise HtD buildings has been marred by several issues. Historically, for example, there’s been a lack of focus on identifying and studying these specific types of buildings, with much of the research skewing towards general energy usage and efficiency. This gap has made it challenging to develop targeted strategies for their upgrade and retrofitting. Also, the identification of HtD buildings has, up until now, been complex and reliant on detailed and varied data that has not been readily accessible or sufficiently prioritised (so far) in energy performance datasets.
Also, technological and economic factors have compounded the difficulty. For example, HtD homes often require more sophisticated and expensive work to make them more energy efficient, which may not be feasible given current technological and economic constraints. This is problematic for not only meeting emissions targets but also for addressing social issues like fuel poverty, where the least efficient homes are often inhabited by those least able to afford their retrofitting.
A New Approach – With The Help Of AI
The new approach developed by the University of Cambridge, uses deep learning to classify HtD buildings. The research team has reported that their new AI-based method for the classification of HtD buildings can achieve an overall precision of 82% on the building level.
The new method uses publicly available data – a dataset of HtD houses (in Cambridge for the test), organised with criteria derived from the Energy Performance Certificate (EPC) which results from detailed inspections of houses. Street view images (SVI), aerial view images (AVI), land surface temperature (LST), and building stock data are also used together for the prediction with deep learning. The AI model at the heart of the new method is also able to reach its classification of buildings by pinpointing the parts of a building which are losing the most heat, e.g. the windows and the roof, and whether a home is old or modern.
What Does This Mean For Your Business?
With so many UK homes being energy inefficient and a major source of carbon emissions, plus the pressing need to decarbonise the residential sector by 2050, yet with identification and classification of HtD buildings being too complex and reliant on data that hasn’t been accessible or sufficiently prioritised (so far) in energy performance datasets, a new method that appears to work is very welcome.
The recent breakthrough by the University of Cambridge in utilising AI trained to identify hard-to-decarbonise buildings using open-source data (a first) is a big step forward that could provide policymakers with a fast and effective way to audit and find out just how many houses they have to decarbonise.
As Dr Ronita Bardhan, the head of Cambridge’s Sustainable Design Group and co-author of the study has pointed out, this new and better tool for targeting energy inefficiency within the residential sector could help direct policymakers identify the high-priority houses, thereby saving them precious time and resources. This new method means that AI could, therefore, provide a way to make better decarbonisation policy decisions, and make serious inroads into reducing the stubbornly high emissions of this sector, thereby also providing a better chance of meeting decarbonisation targets.
Additionally, this progress in AI and building analytics could offer a competitive edge by promoting data-driven decision-making in real estate development, urban planning, and energy policy. As tech companies continually seek to leverage their expertise in data handling, the AI model’s adaptability to identify HtD homes based on open-source data streams such as EPC, SVI, AVI, and LST can be integrated into existing and future tech solutions.
The discovery of this new method (model), which the Cambridge researchers appear confident they can significantly increase the detail and accuracy of over time is an advance in corporate social responsibility. However, it’s only just been tested and the imperative now is to harness its potential and translate it into actionable strategies that yield measurable results in decarbonising the residential sector, aligning with global sustainability commitments, and reinforcing the role of innovative technology in societal advancement.
By Mike Knight
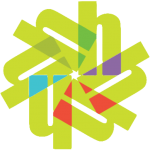